Scientific publications
- [MACHINE LEARNING IN BIOMEDICINE]
- [COMPUTATIONAL BIOLOGY AND TRANSLATIONAL GENOMICS]
- [ENABLE TECHNOLOGIES]
Towards a more inductive world for drug repurposing approaches
Jesus de la Fuente, Guillermo Serrano, Uxía Veleiro, Mikel Casals, Laura Vera, Marija Pizurica, Nuria Gómez-Cebrián, Leonor Puchades-Carrasco, Antonio Pineda-Lucena, Idoia Ochoa, Silve Vicent, Olivier Gevaert & Mikel Hernaez
Abstract
Drug–target interaction (DTI) prediction is a challenging albeit essential task in drug repurposing. Learning on graph models has drawn special attention as they can substantially reduce drug repurposing costs and time commitment. However, many current approaches require high-demand additional information besides DTIs that complicates their evaluation process and usability. Additionally, structural differences in the learning architecture of current models hinder their fair benchmarking. In this work, we first perform an in-depth evaluation of current DTI datasets and prediction models through a robust benchmarking process and show that DTI methods based on transductive models lack generalization and lead to inflated performance when traditionally evaluated, making them unsuitable for drug repurposing. We then propose a biologically driven strategy for negative-edge subsampling and uncovered previously unknown interactions via in vitro validation, missed by traditional subsampling. Finally, we provide a toolbox from all generated resources, crucial for fair benchmarking and robust model design.
REVISTA Nature Machine Intelligence
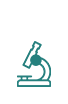
Our authors

